🎧 Listen Now:
What do the best companies differently when it comes to AI?
That’s the question I posed to Asana’s Head of The Work Innovation Lab, Rebecca Hinds.
Rebecca earned her Ph.D. at Stanford University, where she focused her research on transforming organizations through technologies like AI and non-traditional work forms such as hybrid work and remote work.
Rebecca received the Stanford Interdisciplinary Graduate Fellowship, considered one of the highest honors for Stanford doctoral students.
Her research and insights have appeared in publications including the Harvard Business Review, the New York Times, the Wall Street Journal, Forbes, Wired, TechCrunch, and Inc.
Most recently, her team launched a report, “The State of AI at Work,” in partnership with Claude-maker Anthropic.
While I previously covered the report, there was a lot more I wanted to know about what we can learn from the companies leading AI adoption.
Key Insights from Rebecca Hinds
Here’s what Rebecca shared about what AI leaders do differently:
1. Understand the five stages of AI maturity
Rebecca’s framework classifies organizations based on where they are in their AI journey: skepticism, activation, experimentation, scaling, or maturity stage.
According to Rebecca, organizations are at very different stages regarding their AI maturity. Knowing where you stand helps you identify what steps you need to take to progress.
An important way to progress is to look at Stage 5 companies, which Rebecca explained "think fundamentally differently about the relationship of AI with humans."
Make this shift in perspective as it’s crucial for advancing AI adoption.
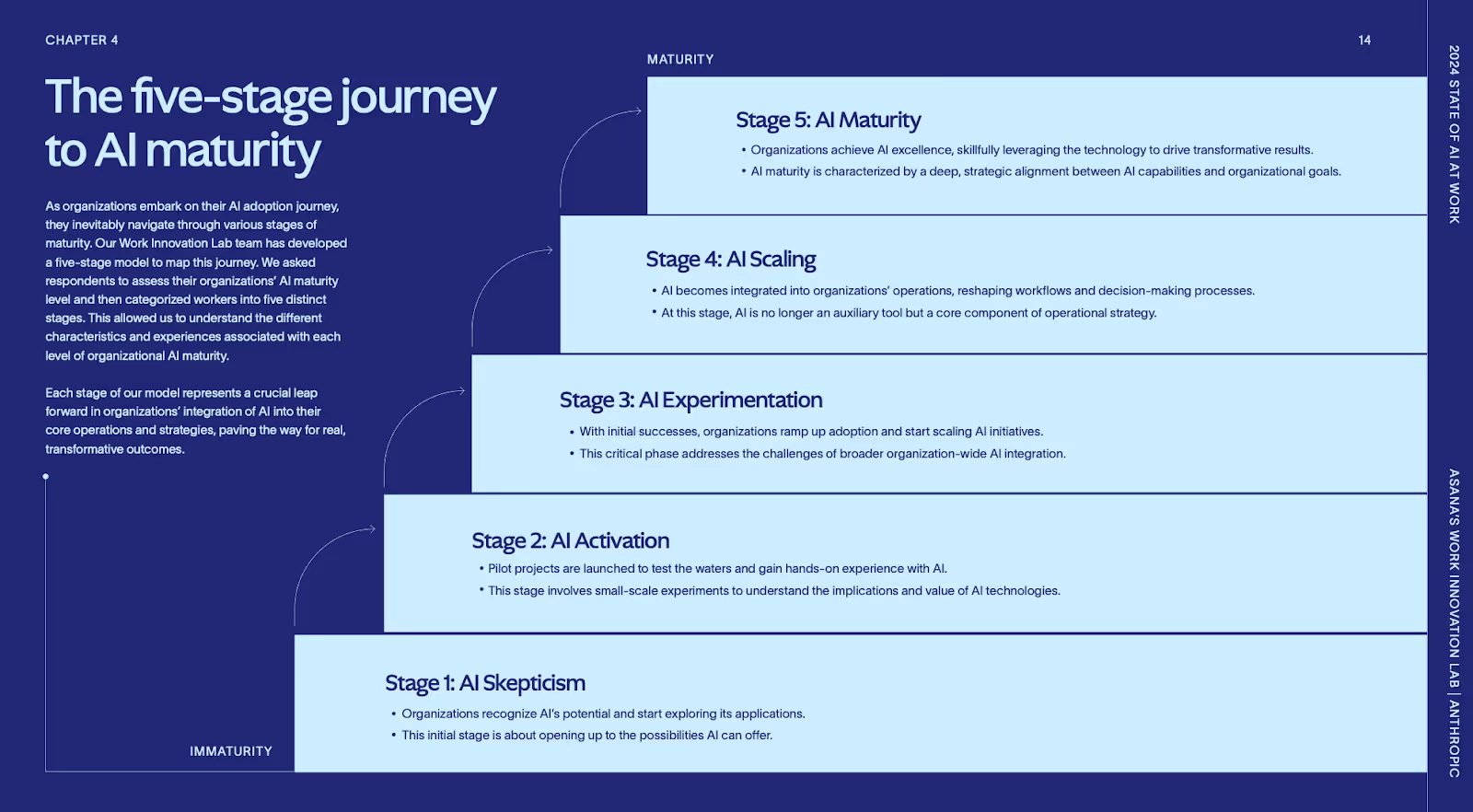
2. Implement the 5 C's framework
To progress toward AI maturity, practice the 5Cs: Comprehension, Concerns, Collaboration, Context, and Calibration.
These elements combined provide a holistic approach to AI implementation.

Rebecca emphasized, "It's multiple different levers that you need to be pulling to make AI work. It's not just having a policy. It's not just framing the technology. It's not just learning and development."
Look at each of the 5Cs to understand your next actions.
Then, look at practical ways Stage 5 companies tap into AI, like always letting AI collaborate on tasks.
3. Focus on the human element with AI as a teammate
Technology alone isn't enough. Successful AI adoption requires employee buy-in and understanding.
As Rebecca pointed out, "You can have amazing, great AI technology... But they haven't thought deeply enough about the human piece. And that's where it breaks down really, really quickly."
Importantly, shift the perception of AI from just a tool to a true team mate for ultimate human–AI collaborations, as stage 5, AI–mature companies do.

In these AI-mature companies, employees don’t ask AI what it can do for them, but look at it much more holistically, opening up more avenues to derive value from AI.
This idea of correctly framing AI was core to Rebecca’s PhD research, and it’s proving true again.
4. Provide tailored, function-specific AI training:
Not every employee will pick up AI equally.
One-size-fits-all approaches rarely work for AI training. Each department may need its own specific guidance, and that also means going beyond just the technology teams. (See our recommendations for the best generative AI courses.)
Rebecca advised, "Marketing needs to have their own training compared to engineering and sales."
Look at the leaders and AI power users within your company that can support teams on this journey.
This targeted approach ensures that each team understands how AI can enhance their specific workflows.
Additionally, it’s crucial to have a resource, a channel, or a person people can turn to when they have questions about how to use AI, as Nichole Sterling and Helen Lee Kupp from Women Defining AI told us last week.
5. Continuously collect and act on employee feedback
As Rebecca shared, “AI can not be a hobby, it needs to be an investment.” But for it to positively impact every business, it has to be implemented correctly.
You also need to assess whether AI is working based on employee surveys.
Rebecca strongly recommended to start “to collect employee feedback. I've seen too many leaders and organizations be in the complete dark about how AI is being adopted, resisted, and embraced in their organizations."
Survey your people regularly and, importantly, show how their inputs are used to make tangible changes in how you adopt and distribute AI.
By implementing these strategies, you can more effectively navigate the complex process of AI adoption, move your team or company toward AI maturity, and reap the benefits of this transformative technology.
Learn More and Lead with AI
Subscribe now to Lead with AI and be the first to hear my conversation with Marlene de Koning, the HR Tech & Digital Director at PwC, about their implementation of Microsoft Copilot.
Want to level up your AI skills today?
- Join Lead with AI, a course and community for executives looking to embed AI in their daily work. Our next cohort kicks off on September 2.
- Sign up for our Advanced ChatGPT Masterclass, a 3-hour live workshop on August 1.
- Take our self-study courses on 10X Better Prompting, Building Your First AI Employee (Free), and 50% More Productive with AI.
Want to get your whole team involved? Check out our offerings for customized AI courses for leadership teams.
If you have any other questions or feedback, or would like to be considered for the podcast, just send me an email: daan@flexos.work.
🔔 Available on:
Transcript:
Daan van Rossum: Very exciting. Last time we talked about work, where we work, when we work, and a little bit about how we work. But today we will focus mostly on that. You just released an amazing study that I've shared with everyone who wants to receive it together with Anthropic, which is an incredible partner about the state of AI. So what's the state of AI?
Rebecca Hinds: Sure. So this is a study. This is our second iteration of the state of AI at work, and it's essentially a deep dive into how organizations are adopting AI, what's working well, what are some challenges that they're facing, and most importantly, what are the companies that are getting it right with AI doing differently than the companies that are getting it wrong?
It's based on a survey of more than 5,000 knowledge workers in the U.S. and U.K., but it also draws on numerous other inputs, including expert viewpoints, to understand how AI is changing or not changing the workplace. And I think the headline is that organizations are at very different stages in terms of their AI maturity.
And what we've done in the report, which I think is truly novel, is classify organizations according to where they are in their AI journey. And what are the distinguishing characteristics of the AI-mature companies versus the ones that are less mature?
Daan van Rossum: We got to talk about that because everyone in our lead AI community is at different stages.
What are the stages that you found through the reports? Maybe we can even talk about how people move up those stages to God-level.
Rebecca Hinds: It ranges from, at the very beginning, the most nascent stage to what we call AI skepticism. And in general, in the workplace, we continue to see high rates of AI skepticism across the board.
But especially among those individual contributors, there's a lot of skepticism in terms of the technology. Will it work? How do I learn how to use the technology? Is it biased? What's really interesting is that we see the level of skepticism and the nature of skepticism change as we move to different levels of maturity, but at those very early stages. (Check out our guide to AI Change Management.)
In Stage 1, which we call the report, we're seeing high levels of skepticism. We then see, at stage two, what we call AI activation. And this is actually where most organizations are; they're most likely to fall into stage two right now. And so, at this point, they're activating their AI initiatives. They're launching pilot projects to test the waters. They're starting to get their hands dirty with AI. But very small experiments and small AI initiatives.
We moved to stage three, what we call AI experimentation. And typically, organizations have seen some level of success with AI. They've piloted it. They've seen some initial success. They're starting to ramp it up across different functional teams. They're starting to think more holistically about organizational-wide AI adoption.
Stage four is what we call AI scaling. So now it's truly true that AI is being integrated into the DNA of the organization. It's a core part of operations. It's no longer ad hoc; it's truly embedded in the strategy.
And then stage five, the pinnacle of maturity, is what we call AI maturity. And this is where organizations have successfully leveraged the technology and multiple different use cases. There's deep strategic alignment in terms of the technology and the business strategy, and AI is essentially working well for the organization, and they're starting to see those results.
Daan van Rossum: Has anyone reached level five yet?
Rebecca Hinds: There are a few companies, so definitely there are some, and I think it's important to be able to identify them and learn from them as well.
Daan van Rossum: And that's probably the LLMs themselves, who were early enough to adopt all of it.
Rebecca Hinds: It's always this combination of technology and the human piece. And I think the companies that are getting it right with AI right now are thinking very carefully about both components. You can have amazing, great AI technology. And we've seen this in some cases where companies have invested in the latest, greatest AI technology.
But they haven't thought deeply enough about the human piece. And that's where it breaks down really quickly in terms of whether you have great technology. You don't have the adoption of that technology by your people. And then there's little benefit to the technology if it's not truly ingrained in your organization and people aren't using it.
Daan van Rossum: Yes. Multiplication by zero is still zero. So you do need people using the technology, and that must be part of how companies level up. So what are some things that you're seeing in terms of what works? To get everyone aboard the AI train.
Rebecca Hinds: So another part of the report is what we call the five C's. And so, essentially, as you move from stage one to stage five, what's interesting is that companies differ across these five key dimensions that we call the five C's. And so their comprehension is the first one. So essentially, we see at stage five that, perhaps not surprisingly, employees within organizations have a much deeper comprehension of AI. So they know how it works there. They've participated in training. They've leveled up their skill sets. They have that level of comprehension.
The second is concern. And this is an interesting one because what we see is that we all have some concern about AI. It's inevitable that we do, but the nature of our concern differs depending on what stage of maturity our company is at, and so at stage one, we talked about skepticism. That's what we see. We see a lot of skepticism. And we see that skepticism is focused on that first sea of comprehension. So at stage one, most employees are focused on: are they able to learn new skills? They're concerned about where I go to learn AI. How do I uplevel my skill set?
Whereas at stage five, the concerns center more on ethical and responsible AI, especially what we and lots of others call interpretability. Can I interpret the results of the AI model? So companies at stage five that are doing it are really investing in tools or capabilities that enable employees to interpret the results of the AI model.
The third, which I think is also fascinating, is collaboration. So we see that at stage five, companies are fundamentally thinking about the technology differently in terms of the relationship of AI with humans. And what we see in particular is that at stage five, employees are much more likely to see AI not just as a tool but as a true teammate that they're working with.
In my PhD, I studied quite deeply this idea of technology framing and how, throughout history, we've seen that. How people frame technologies matters deeply for how they end up being adopted. And so this was something that I was super fascinated by—how this mindset shift from tool to teammate really can change the way you interact with technology.
And we see that's the case. We see that when employees view AI as a teammate, they're much more likely to think holistically, not just about what AI can do for them as a tool, but how they can work with the technology as part of these human-in-the-loop workflows. It seems like a trivial mindset shift, but we see it in the research, and we're just doing a study we're about to release in a couple weeks on the importance of this AI mindset, and it matters. It has an outsized impact. So that's a really important one.
Then the fourth one is contact. What does your organizational context look like that you're bringing AI into? And here's where we see a lot of opportunity for improvement across the board. We consider context to encompass the policies you have around AI, the principles, and the guidelines. And it's shocking how few companies have any sort of policy, have principles, or have guidelines in terms of how you use AI. That's what we see at those stage five companies. They not only invest a lot of time into developing those foundational elements, but they do it early.
And then the final one is calibration. So we know that we need to be assessing the results and measuring the impact of these AI tools. And at those stage five companies, they're much more likely to be evaluating the success of their AI initiatives compared to stage one, where it's much more sporadic, involving unstructured attempts to measure the impact of AI.
And so what I think is important about this framework is that there are multiple different levers that you need to be pulling to make AI work. It's not just having a policy. It's not just framing the technology. It's not just learning and development. It's a combination of these different factors. And I think the 5C framework helps to provide some of that scaffolding in terms of the elements that you should be thinking about as you work towards AI maturity.
Daan van Rossum: I love this framework because it is so sexy. It's so clear in terms of where we should get started, but it sounds like a lot of work. So you're already investing in the technology.
Then you have to invest in all of these five C's. At what point do companies start to say that we don't even know if this is going to be worth it? The final C is: do we actually measure and see if it contributes to our company's productivity or revenue?
Where do companies make that assessment of whether this is all even worth it?
Rebecca Hinds: AI cannot be a hobby. It needs to be an investment, right? This is a transformative technology. It has vast potential. I think there's no question that it has the potential to positively impact every business if implemented correctly. But it can't be something that you invest 5% of your effort into.
It needs to be an investment right up front. Now, I think it's important to start small. And that's where feedback, results, and iteration really matter. I think the most important, if I had to say one initiative that an organization should take as they're going on their AI journey, is to collect feedback.
We see that far fewer companies in that stage are actually collecting feedback from their employees on whether AI is working or not. Those stage-five companies are much more likely. We see that over 90% of them actually collect feedback, whereas in stage one, far fewer of them actually collect the feedback.
That's essential because your employees are very well in tune in terms of whether AI is working and what challenges it's solving on the ground. I think the biggest struggles I see companies face right now are: one, I see that's relevant to what we're talking about here: companies try to overengineer the metrics that they're using to assess AI.
And they try to cover everything. They try to develop a scorecard that encompasses 20 metrics. They try to overengineer the evaluation of success. And I think that's where things can spiral really quickly at the earliest stages. I think the most important thing you can do, in addition to having clear guidelines and a perspective on the role of AI in your organization, is to collect feedback from employees.
Understand, is it working? Are they able to learn how to use the technology? How often are they using the technology and not assuming that just because the technology is technologically sound, it's going to be adopted in your organization?
Daan van Rossum: Another thing that really jumped out to me in the report to me is very alarming actually is also the gaps between individual employees. So some people, depending on the stage of the company, maybe, but some people. Getting it, wanting to try it out, and starting to really learn it. And then some people are not really getting into it.
I'm really worried that's going to create a gap. That's only going to widen because the longer you wait to learn it, the more difficult it's going to become to get into it as the technology develops way quicker than anything we've seen before. So I started in the report, but maybe any kind of context around, what is that gap? What does it look like? And also, what can companies do to make sure that everyone goes along, at least at a somewhat similar pace?
Rebecca Hinds: I think the gap is especially concerning because we see it across multiple dimensions, but we especially see it across the levels of hierarchy, such that individual contributors are much less likely to use AI and feel enthusiastic about using AI compared to senior executives.
And they're also disadvantaged in so many other ways in terms of having the resources to pay to attend conferences or AI courses. And so, I do think there's a real fear of the gap widening. I think one of the biggest pitfalls that organizations are falling into is that they assume that they can develop one-size-fits-all training.
And for AI, that's persona-specific. So functionally specific. One-size-fits-all training rarely works. I think it can work for some of the principles, ethics, and safety-type training, but it can't work for the day-to-day. How are you truly going to embrace this technology as a core part of your workflow? It needs to be functionally specific.
Marketing needs to have its own training compared to engineering and sales. And so we see often that a lot of the training is focused on the technical folks in the organization, the engineers as well. And they're arguably also the most able to learn the technology on their own.
And so you see these multiple different dimensions of these potential gaps widening. And I think it's really important to invest in that learning and development at the very early stages.
As you said, this technology is evolving so quickly, and I truly believe the more we can learn with a fundamentally flawed technology, the more important it is because the only way to keep up with these changes in models and capabilities and all the different components of AI is to learn with them and learn how they're evolving.
And right now, I think there's a big wait-and-see mentality within a lot of organizations: let's roll out the technology. Let's see if it has an impact. And then we'll invest in training. And it just never works well when it's that progression.
Daan van Rossum: That's why it's nice to look at something you mentioned in the report around these level five organizations to see how they're doing it.
Because, as you can see, people treat it as a coworker, not as technology embedded within their workflow. Typically, those power users have way more use cases for how to use AI than someone who's more of a novice, right? So you're looking at those organizations; you look at those examples. Okay. That's something I can follow. Then still again, like people listening to this, maybe at all kinds of different levels of that adoption curve.
What are some fundamentals that anyone can implement? I'm just thinking about something practical, like training by team, right? okay. That sounds fantastic. But for an organization, now we need someone to train the marketing team. Now, we need someone to train the sales team, right? Like, how do we practically get started, or how do we practically continue on that evolution?
Rebecca Hinds: I think a big first step is actually having a philosophy around the role of AI within your organizations.
This is something that we did very early at Asana. We're very thoughtful about our principles around AI. And we talked a little bit before we went live about why we partnered with Anthropic and how our principles and overarching viewpoints on AI were very much in alignment. That's something we thought about very early in terms of how we view the role of AI alongside humans, and I think that has so many different positive impacts on your organization.
It gives employees confidence. What is this technology going to be used for? What is the purpose of it? The purpose of it isn't to take my job. It's to amplify my human skill set. And I'm still going to be in the driver's seat because AI is playing a supportive function or a teammate function.
And I think having that clarity is, in a sense, a type of training. You're training through having a resource that employees can point to and turn to as they're navigating this new technology. I do think training can be expensive. I do think the investment is worth it for organizations right now.
I also think there are power users in every organization I've seen where they're very willing, in my experience, to share their learning, share success stories, and share different initiatives that they've found to be helpful in their learning journey. We did a fantastic workshop a couple weeks ago with a group of Stanford students who came to learn, and we had some of our internal AI experts teach them how to write a prompt. And these were all employees that weren't trained in delivering AI training but had deeply invested in the technology themselves in terms of upleveling their skill sets and were able to lead a successful session.
So I think that. You can be creative in how you go about training, but it needs to be a concerted effort. And I think the investment is absolutely essential.
Daan van Rossum: Perhaps even combine them. You can say we're going to write a charter for the company. This is the role of AI, not just in our product and in the future of our business, but also internally in how we're going to use it. What is the role? What are some of the ethics—maybe some of the core principles around that? And then you have these enthusiasts that you should definitely tap into, right? Because people who get this really get it. And I think they love to share, right? They're very innovative and very forward-thinking.
They would love to share it with other people, get them involved, and mobilize them. What are some practical things that you've done, even in your team, in a smaller scheme, or maybe in the organization? What are some practical things you've done to get everyone on AI? And I'm assuming your journey started quite a bit before most people's journeys with AI.
Rebecca Hinds: I remember one of our first reports, and this would have been about two years ago when we started the work innovation lab. We were a very scrappy team. We didn't have a lot of resources, and we started to use AI very early to generate the images for our reports. So we weren't paying for the expensive stock photos.
We were using DALL-E to create images for these reports very early. And I remember. Going through the approval processes to be able to use the technology internally. And it was like, It was so new. It was so new that you could do this—that you could create images using AI for reports. And we ended up saving a lot of money very early. And ever since that point, I've really encouraged my team to use AI for everything possible. At least once, try it for everything possible. And I think that activity is where you're truly immersing yourself in the technology, because that's how you understand whether AI can be beneficial or whether humans are still better suited to perform a given task.
So my team uses AI deeply. And a lot of our team is really technical. So we're also developing our own models. And a big part of our work involves working with customers to help them understand how they're collaborating and how those collaboration patterns lead to certain work outcomes.
And my team, led by Dr. Mark Hoffman, who's led a lot of this work, has developed these deep AI models and neural networks so that they're able to deeply understand the specific collaboration patterns within an organization and how they're contributing to different work outcomes. So what I've seen as well is that in every company, there was an initial push to, okay, we're going to invest in AI, we're going to have these internal trainings, and I've seen that taper off.
While recognizing that there is that natural paper effect that often happens with new technology, I think we've made a concerted effort to keep trying new use cases, not rest on our laurels in terms of what we know AI works for, and continue to push the technology and see where it does and does not work.
Daan van Rossum: I love the idea of just using it for everything possible. I think Ethan Mollick always calls that; always give AI a seat at the table. Prove yourself wrong, right? Like, just try AI and see if it works for that. And then you may be surprised, or maybe not so surprised, that it works well for certain things and doesn't work well for other things.
Rebecca Hinds: Because the technology is evolving so quickly too, it might not work today, but it might work tomorrow. And having that constant iteration, I think, is so important.
Daan van Rossum: I was going to say that's the other element of this. We sometimes see this in discussions on X, right? Oh, what tool can I use for this or that? And you should use that. Oh, I tried it three months ago. It didn't work. Yeah. But everything has changed already. So there's so much happening.
Do you have any rituals or any things that you've baked into your way of working to get people to keep exploring new tools? Also, maybe share and maybe highlight successful use cases to keep that habit of constantly trying things with AI going.
Rebecca Hinds: I think it's a multi-pronged strategy. So I do think we have several Slack channels both within our team at the work innovation lab as well as at Asana, where we're constantly sharing what we're learning. What are we using AI for? What are some success stories? And I think that constant sharing, sharing failures, and sharing where AI has fallen short of expectations are also important.
I think having some sort of channel to do that is really important. I think you should have a list of people or publications that you constantly follow and keep tabs on. I think you're one for me, for sure, Daan, where I'm constantly turning to your posts and your newsletters to learn the latest.
I think Charter is another one that I love. I think they produce amazing content. And if you have three or four pieces, I think it can get overwhelming to try to keep up with everything. But if you have those go-to sources, that's often enormously helpful for me. I am still very deeply embedded in the research community and the academic community.
So I'm always keeping abreast of those developments as well. And I think for every person, it's going to be different. I do think you should have a domain-specific type of resource or resources, and if you're a marketer, having those domain-specific ones is important too.
And I think conferences—there are a lot of great, both virtual and in-person, conferences on AI. I know VentureBeat and Transform, and it's often super beneficial to not only go and listen to the sessions but also talk to people and have those conversations in the hallway where you're learning things that you might not be able to learn from a stage presentation, but you're truly interacting in a way that gets into the nuts and bolts of how people are actually adopting the technology.
Daan van Rossum: I had AJ Thomas on recently, and he said curiosity is going to be our biggest superpower. It's developing very quickly, but we cannot just let it wash all over us. We need to actually be curious and dive into it.
I also really liked that point about being connected to something in your industry, because, like you said, this is all going to be 10 times more valuable if you get the application within your particular field—how to use AI in marketing, AI in sales, and AI in leadership. That learning community is so powerful to be a part of because you can ask stupid questions, get recommendations, and solve problems outside of what you would do within your own team or company. So I think all of those are valuable.
So I'm going to ask you three rapid-fire closing questions. Number one, what is your favorite AI tool besides Asana?
Rebecca Hinds: I'm going to say Asana, because I think, and Asana, we use open AI and philanthropics, so talking about multiple tools really is at the foundation. But I think Asana just has so many different AI use cases that I've actively used and embraced as part of my role.
My favorite is what we call smart status updates, where we are very good at documenting at Asana, and that documentation has historically taken a lot of time. And we've just passed the end of June. At the end of the month, I used Asana AI to generate all the status reports for all my projects.
Every research report reports on the state of AI. I said, Ask AI for a summary of this project, where it showed up in the news, where it showed up in various places, and you can always edit it. And I often always do at the end of a status update, but it saves me hours of time every month.
And I think that tangible benefit is something that I'm grateful for and impressed by.
Daan van Rossum: Okay. That's totally worth it as a favorite AI tool.
Then, maybe one unique use case of how you're using AI in typical daily workflows.
Rebecca Hinds: It's super different, super impactful, where he's developed AI models to essentially be able to evaluate a company's propensity for innovation and various other work outcomes just by how they're collaborating on Asana.
When I think about the pinnacle of using AI at work, it's to improve how we work together. It's not to boost individual productivity. It's to truly enhance teamwork and organizational effectiveness. And that's where we're headed, and with the Work Innovation Score, being able to assess AI and learn what the best companies do differently is both unconventional and incredibly exciting.
Daan van Rossum: After all of this, a lot of to-dos, and a lot of calls to action, what's one thing that business leaders should do tomorrow to get ahead in AI?
Rebecca Hinds: I think it's time to start collecting employee feedback. Every organization these days does some sort of pulse survey or engagement survey. It's very easy to embed a few questions. And I think I'd encourage you to base those questions on the report and those different seasons and stages of maturity. It's not a heavy lift. And I think it's something that every organization can do, and every organization is going to learn from how employees are embracing, or probably, moreover, resisting, technology right now.
Daan van Rossum: Amazing. So even with all this innovation, it still comes back to the fact that you have to listen to people. Rebecca, thanks so much for being on.
Rebecca Hinds: Thanks so much, Daan. It was fun.